With consumer viewing habits changing rapidly, traditional methods of labelling and logging metadata no longer seem to be keeping pace.
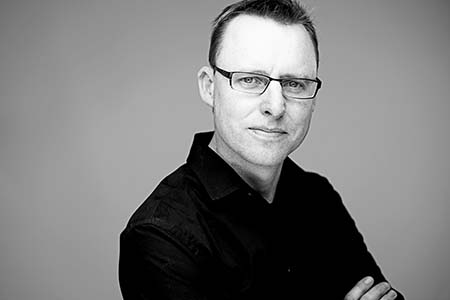
With consumer viewing habits changing rapidly, traditional methods of labelling and logging metadata no longer seem to be keeping pace. Samuel Conway proposes a new integrated method that combines the power of AI and machine learning, for faster content discoverability from multiple sources.
In the broadcast market, where so many applications are mission-critical and audiences are ever more difficult to retain, every position in a broadcast organisation, be it producer, researcher, editor, content programmer or marketer, entails dealing with a tsunami of unstructured digital media and data which must be scrutinised to make decisions, sometimes at very short notice.
Broadcasters are awakening to the age of data overload, as cloud processing facilitates even more sources of information and delivery platforms, while social media, IoT (Internet of Things) devices and smartphones bring challenges of their own.
The advantages of more data are clear, of course. It brings the opportunity to provide more content and create more audience satisfaction and engagement. However, it also brings with it all manner of issues when it comes to big data structure management and analysis. How does all this data easily translate into meaningful information that allows broadcasters to provide more engaging, timely and relevant content to audiences and subsequently bring in more revenue?
The structured data that traditionally makes up asset management systems comes with metadata that has a defined structure or taxonomy that rarely changes over time. Standard keyword and search interfaces are then used to access that content, so it can be used within a production or broadcast system.
However, new technologies like OTT and social media have dramatically changed the way we consume media, and with the current process of labelling and logging metadata, it is impossible to anticipate future uses for content. And unlike structured data, these large, disparate sources of volumous unstructured data must be processed and analysed before they can be quantified, understood and then used effectively.
Combining AI and Human Sentiment
Despite being very good at understanding the messy, unstructured nature of the world, the processing performance of the human brain cannot scale at the rate that unstructured data is growing.
Broadcasters all know that a content library remains worthless without some means of knowing what is in the archive. This means they need to access much more than titles and an outline of content: as automation becomes ever more prevalent, they need metadata that can provide actionable insight.
The recent avalanche of new data means a new way of thinking is needed when managing a digital archive, one which allows broadcasters to anticipate the arrival of the next disruptive technology or cultural movement. This requires that manual loggers be as exhaustive as possible in the metadata that is captured to anticipate future needs.
Enter artificial intelligence (AI) and machine learning. These have the capability to consume large amounts of data streaming in from varied sources and, more importantly, to make sense of it all. By leaving tasks like face and logo detection, sentiment analysis, pose detection and metadata extraction to AI, humans can step away from the repetitiveness of manually annotating data, to make better use of their higher cognitive functions.
This is especially important in the media industry, where the impact of the content is very subjective by nature something machines do not handle well. Through the large-scale processing of many media files in a short space of time, AI can extract vast amounts of metadata.
Machine learning is increasingly adept at classification tasks such as identifying sentiment, emotion, pose and activity. It can also upscale video, remove objects and add colour to video. This makes it especially useful for enhancing existing archives where little or no metadata exists. The best part is that it can be run 24×7 and can be upgraded to new and better machine learning algorithms as they appear.
A Paradigm Shift in Search
Traditionally, exploration and discovery of data have been facilitated by search. Until now, the concept of search has offered one user experience: a single text box where a word or phrase can be typed, with the results all looking the same, i.e. 10 to 50 links in a series of pages.
The idea of making big data immediately comprehensible to the human eye, through dynamic interactive visualisations, is a new approach that offers exciting possibilities to the broadcast industry. Engaging both machine learning and human intuition is the key to dealing with this data overload, and is bound to empower people to make decisions more quickly.
Sectors such as sports, retail and medical research already use visual exploration technology, and we believe it will also transform how broadcast organisations make judgement calls on relevant content, quality and processes.
Collating the masses of unstructured visual data from disparate private and public systems into a single platform and transforming it into valuable and purposeful visual information represents a paradigm shift in search. If an entire collection of information is presented in a single field of view, users can take in the full scope of the data all at once. There is no need for paged interfaces, so users can instantly see the shape of the data and leverage the innate abilities of the human mind to identify patterns.
Delivering and Enriching the Moment
The tools currently being used by broadcasters to make big decisions are either aggregated business intelligence (BI) and analytic tools that only deal with numbers and text, or asset management software with no analytics element. Businesses tend to employ multiple sets of teams with specific skills to make sense of all the data at their disposal, but this task can be insurmountable and costly.
So, what if a unique algorithm could be used to draw all of the information from a broadcasters structural databases, including MAM systems, metadata, API, statistics as well as unstructured data such as social media and live data platforms, into a single, highly visual user interface using proxies and webhooks?
This would provide a highly interactive way of looking at content. Using a customised faceted filter system, producers, researchers and editors could analyse all the data and dynamically position each individual item on a single screen. They could figure out what their audiences want to see in that moment, without having to work through lists or search engines or have a researcher do any groundwork before a programme airs.
What if a unique algorithm could be used to draw all of the information from a broadcasters structural databases, including MAM systems, metadata, API, statistics as well as unstructured data such as social media and live data platforms, into a single highly visual user interface using proxies and webhooks? It would provide a highly interactive way of looking at content
During live sports coverage, media or metadata could be cross-referenced with live social media using visuals such as graphs, scatter plots and media tiles, then lassoed to see instantly which players are being tweeted or posted about in real time. The broadcaster would then know exactly what its audience wants to know at that exact moment.
If the programme editor needs to display statistics on a goal scorer, they would not only access the players performance stats, but historical facts, images, video and real-time social media influence too which could also be called up along with a collection of similar players over history, or a collection of similar goals. The possibilities would be endless, as would the number of comparisons that could be drawn in the blink of an eye.
By converging both content search and analysis on the same platform, broadcasters could save time starting from scratch with their archive or asset management system by dropping this type of platform on top via APIs which would then tap into the existing archive and live systems, to provide a new level of insight and the ability to dive into and find relevant content in the moment. Such a concept would unlock hidden or previously unutilised content and make it easy for someone without a technical background to embrace AI and explore that content quickly.
Unleashing the power of AI and transforming volumes of data to make it immediately comprehensible to the human eye means broadcasters can build even richer and more relevant content, despite the new dawn of big data.